
Use case 5: Object identification and related searches The back-end metadata includes pronunciations of words in different languages, which are identified and matched with the voice command provided to interpret the commands. which helps in identification of the face. Similarly, programs are designed to identify facial attributes such as retina scan, nose, etc. This system runs on a deep learning technology which is able to distinguish between languages and understand them effectively based on metadata sets fed into the system. Wipro’s customers have adopted audio recognition and identification of dialects to understand the verbal commands and act upon them as instructed. The data labelling services have now expanded beyond just text and images. Use case 4: Natural language interpretation, speech & facial recognition The more comprehensive and extensive the dataset is, the better the performance level of the technology, and closer it gets to human reaction to any changes in the vehicle’s environment. However, for it to react and function properly in a real world environment, it requires a back-end database which helps the vehicle to immediately identify the surrounding objects, project its trajectory, and take corrective course correction decisions to prevent collision and follow the traffic rules effectively. Self-drive cars are fast taking over the automotive market. Use case 3: Replacing human actions in self-drive cars Similarly, uniquely identifiable facial and bodily recognition has taken biosecurity to the next level.
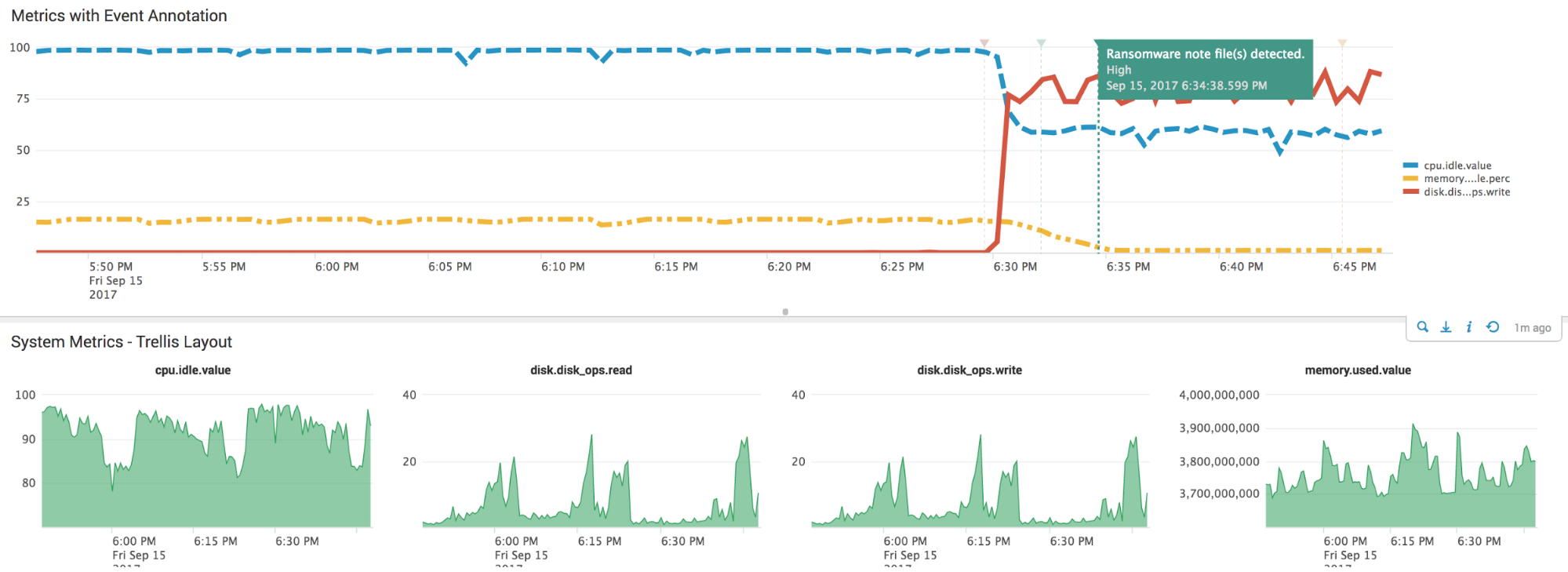
Speech recognition technology has made content more accessible to people with visual and hearing impairments. Today, chatbots and voice assistants have become popular, and are trained to have more human-like conversations with the user. This includes metadata tagging of articles like text, video results, audio, images search, etc. With the help of data annotation, high quality search results are obtained for search queries, as the data is tagged to possible search keywords accurately, which is then sequenced appropriately based on the relevance depending on the search query. Millions of such annotated data then serve as reference point for the AI, thus helping in significantly faster and accurate medical diagnosis, saving multiple human lives in time. Research by Johns Hopkins has indicated that diagnostic error related payments amounted to $38.8 billion between 19, while the misdiagnosis related significant permanent injury or death annually ranges from 80,000 to 160,000 patients in the US region.ĭoctors and medical institutions use data annotation services to create bounding boxes around medical examinations in medical imaging, ultrasound scans, MRI scans, etc. There are numerous examples across industries where the use of machine learning coupled with data annotation capabilities have drastically improved the outcome with much lesser turnaround time as compared to human intervention. The global data annotation tools market size was valued at USD 494 million in 2020 and is expected to expand at a compound annual growth rate (CAGR) of 27.1% from 2021 to 2028, according to Grand View Research. Hence, the accuracy and comprehensiveness of such data sets hold paramount importance. But machines only act according to the underlying parameters set by humans. With the fast adoption of AI across industries, data annotation has also taken a front seat in driving this shift to rely on deep learning solutions and decision making. Data annotation activity is the primary bridge between raw data and AI/ML human-like actions.īenefits and importance of AI- data annotation Experienced GIS experts work on curating and maintaining such data on a regular basis within the client systems without disrupting their daily operations. This data must be guarded and updated and be accurate and precise enough to help the underlying technology to work efficiently. essentially the eye of the camera, depending on the industry, purpose and use cases.
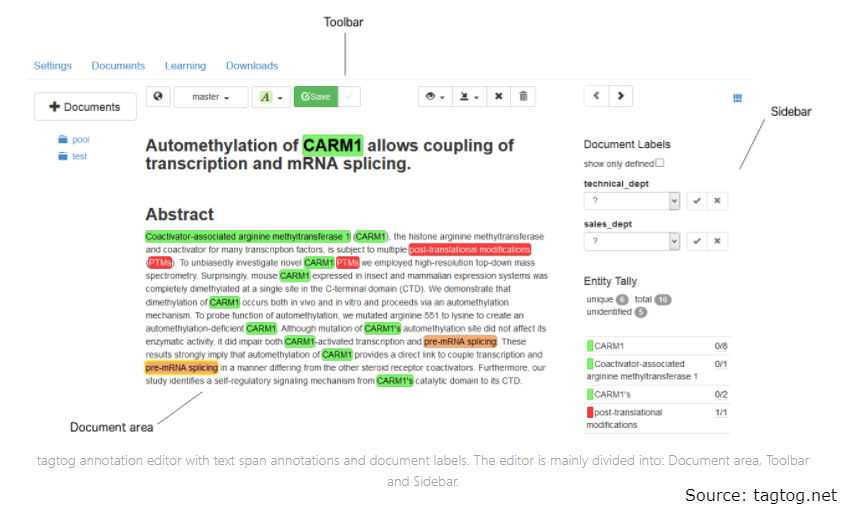
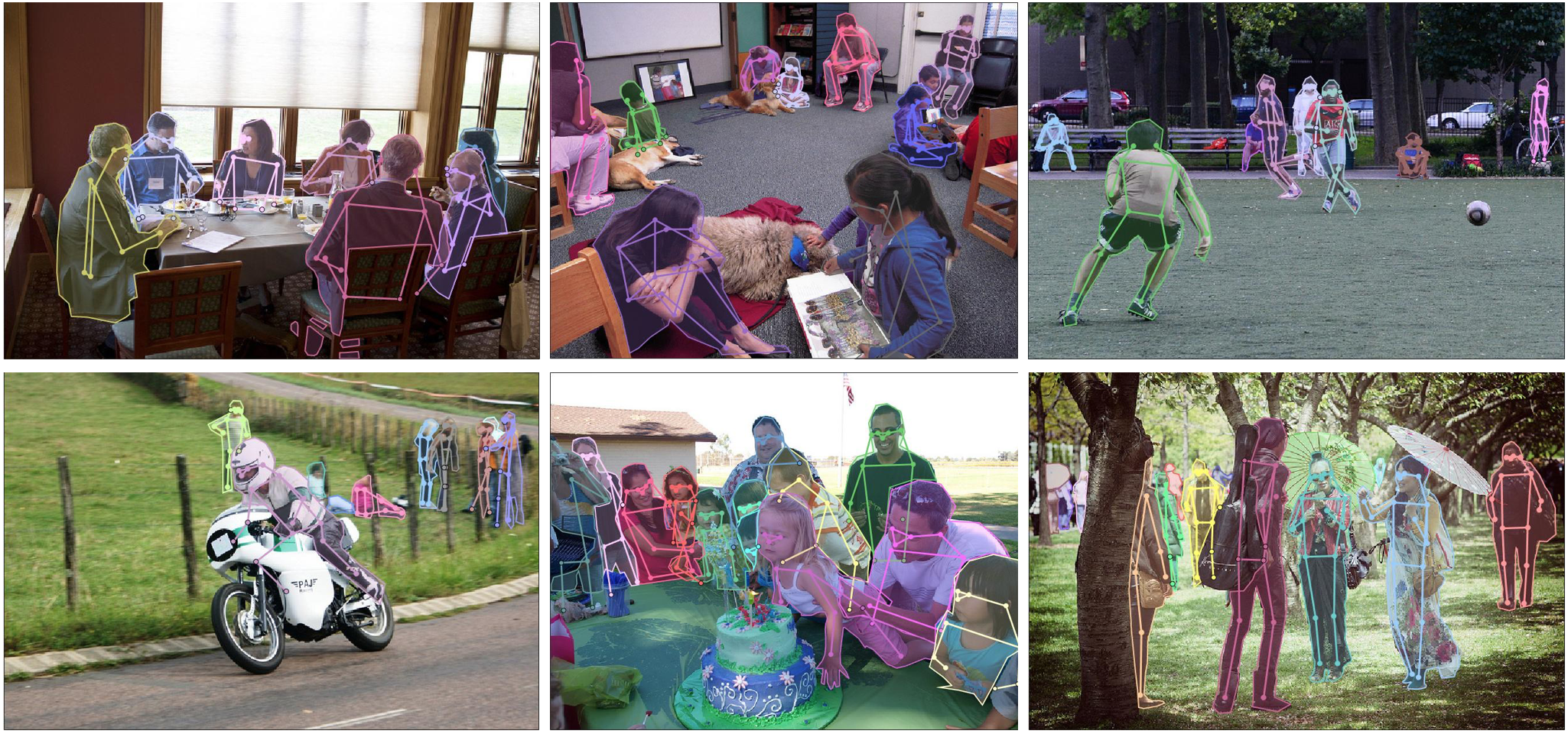
This data can be in the form of images, text, videos, audio, simulations, real time IMS, sensor data & image curation for ML, perception modelling, logical simulations, event testing and triaging, etc. Interestingly, these technologies rely on the data in the back end to be able to make better decisions and gradually improve their artificial thinking power. The delegation of responsibilities has expanded beyond fellow humans in 21 st century across industries. With the increased popularity of artificial intelligence (AI) among leading corporates, decision making is fast slipping in the hands of machines and AI/ML technologies rather than humans.
